Abstract
Computerized clinical decision support systems (CDSS) are increasingly being used to facilitate the role of clinicians in complex decision-making processes. This systematic review evaluates evidence of the available CDSS developed and tested to support the decision-making process in primary healthcare for stroke prevention and barriers to practical implementations in primary care settings. A systematic search of Web of Science, Medline Ovid, Embase Ovid, and Cinahl was done. A total of five studies, experimental and observational, were synthesised in this review. This review found that CDSS facilitate decision-making processes in primary health care settings in stroke prevention options. However, barriers were identified in designing, implementing, and using the CDSS.
Keywords: Clinical decision support systems, electronic health records, prevention, primary health care, stroke, tools
Introduction
A clinical decision support system (CDSS) helps healthcare institutions to analyze data from electronic health records (EHRs) and make recommendations to physicians by sending prompts and reminders in real-time1. CDSS systems can be divided into three general types: diagnostic, patient management, and alerts and reminders1. The computer-based CDSS analyses EHR data and, as a result, transmits alerts and prompts to assist health care practitioners in adopting clinical guidelines throughout the provision of care. A CDSS platform leverages patient data to give health care practitioners with individualised assessments and interventional recommendations based on scientific evidence1,2.
For example, CDSS can incorporate a reminder for overdue screening services for stroke risk factors2. This enables the system to use patients’ history, results from clinical tests, and symptoms to assess their risk of having a stroke or one recommending treatment options to be considered by the healthcare provider depending on the patient’s risk of stroke2. A CDSS tool may also suggest lifestyle modifications that the physician and patient can explore together2.
This systematic review aims to identify available decision support systems or tools that have been developed and tested to support the decision-making process in primary healthcare to prevent stroke in primary care settings.
CDSS improves the screening of the risk factors that indicate the occurrence of a stroke3, 4, 5. The significance of using CDSS to improve stroke prevention is justified by the number of fatalities and morbidities due to stroke in the recent past. Saini et al. (2021) report in the analytical report of global epidemiology of stroke that cases have risen from 1990 through 20166. In 2016, 80.1 million cases of stroke were reported worldwide. On gender-grouped data, there were 41.1 million (38.0–44.3) cases among women and 39.0 million (36.1–42.1) among men6. Feigin et al. (2017) reported that over time, the burden of stroke, that is the resultant health complications after stroke, has risen since 19907. Even in small populations, the prevalence of stroke is observed at 2368 and 2967 per 100,000 (crude and age-adjusted respectively)8. This can be reflected in the global stroke burden as reported by the World Stroke Organization (WSO) in the 2022 stroke fact sheet9. Between 1990 and 2019, the fact sheet reported rises of 43.0 percent deaths from stroke, 70.0 percent increase in incident strokes, 143.0 percent disability-adjusted life-years (DALYs) lost, and 102.0 percent prevalent strokes)10. These numbers have climbed beyond 100 million cases annually, thus increasing the risk of patient death.
These increased risk levels demand more preciseness to help primary care providers prevent the occurrence of strokes and other fatal cardiovascular diseases. Computer-aided diagnostics and detection tools are therefore getting appreciated every day in the medical domain to improve the chances of patient survival in the most critical events.
Studies and other investigations have looked at the possibility of quality-of-care improvements by looking at clinical outcomes related to morbidity and mortality for numerous health conditions. Bright et al. (2012) reported that by looking at 148 randomized controlled trials (RCTs) assessing the implementation of CDSS systems, healthcare processes seemed to improve preventive, diagnostic, and prescriptive healthcare processes11. However, there was not enough evidence to warrant the conclusion that the clinical outcomes improved.
Additionally, the review focused on the application of CDSS against multiple processes such as cancer screening, immunization, and cardiovascular disease prevention. Three years after this review, Njie et al. (2015) carried out a review seeking to fill the gaps of evidence left by Bright et al. (2012). In their findings, Njie et al. (2015) confirmed the results of Bright et al. (2012) with an addition of the significance of CDSS in disease treatment12. The specificity of this review was lacking hence the recommendation that further research should concentrate on practice-based settings to better understand barriers encountered by implementers and challenges posed by generic, mass-produced EHR software not tailored to practices’ needs.
Following the recommendation by Njie et al, (2015), this systematic review looked at the primary care setting and evaluated the performance of CDSS in preventing a single clinical outcome (first stroke). Therefore, the article is a systematic review carried out to identify the available decision support systems or tools that have been developed and tested to support the decision-making process in primary healthcare to prevent stroke in primary care settings. It also identified the barriers facilitators encountered during design, implementation, or using CDSS and summarized the core aspects of the decision aids.
Materials and Methods
Systematic Review Objectives
- To identify available decision support systems or tools that have been developed and tested to support the decision-making process in primary healthcare to prevent stroke.
- To identify any barriers or facilitators encountered during design, implementation or using CDSS.
- To summarize the core aspects of the decision aids.
Key terms: Clinical, decision support system, tools, computerized, electronic health records, prevention, stroke
Search Strategy
The search of this systematic review started March 1, 2021 and finished in September 2021 and includes papers from the initiation of each database to 2021. The search was carried out using key terms and search terms outlined in Table 1. Keywords like clinical decision support system, tools, computerized, electronic health records, prevention, and stroke were used to build a search strategy. The databases of Web of Science (1970-present), Medline Ovid (1950 to present), Embase Ovid (1980 to present), and Cinahl (1981 to present) were searched. In addition, a manual search was conducted of the references to identify any missing papers. The search was repeated just before starting the analysis stage to avoid missing any new studies eligible for inclusion.
Inclusion and Exclusion Criteria
Inclusion considered studies focusing on the prevention of stroke in primary care using CDSS. These studies had to be original papers in English that implemented CDSS in primary care settings with the aim of preventing stroke. A study was only included if patients were adults who had visited primary care. Clinical management in the study had to have used CDSS systems, tools, or applications to manage these adult patients' risk factors.
Study Selection & Data Extraction
The search results were uploaded to Covidence, a web-based program designed to manage the screening process in systematic reviews. Titles and abstracts were initially screened by two reviewers (SA, ST) independently. Then, full paper evaluations were conducted by the two reviewers independently. In case of disagreement, the papers were sent to a third reviewer for solving the disagreement. Data extraction of the study type, country, settings, methodology (population and sample size, sampling methods, data collection, and data analysis), intervention, and outcome were carried out. These data were extracted using an Excel spreadsheet. Characteristic of studies and results and relationship was systematically summarized by narrative synthesis.
As shown in the PRISMA diagram, records Identified from the databases based on our search strategy were 159, the abstract and title screening was done for 141 studies, 18 duplicate papers were removed. One hundred and forty-two studies were screened by two reviewers independently where 116 studies were excluded, and only 26 were included for the full-text screening. Only five papers were included in the systematic review. The other 21 papers were excluded because of the following reasons they were not primary papers, they were not relevant to primary care, or they were not related to the prevention of stroke.
Figure 1
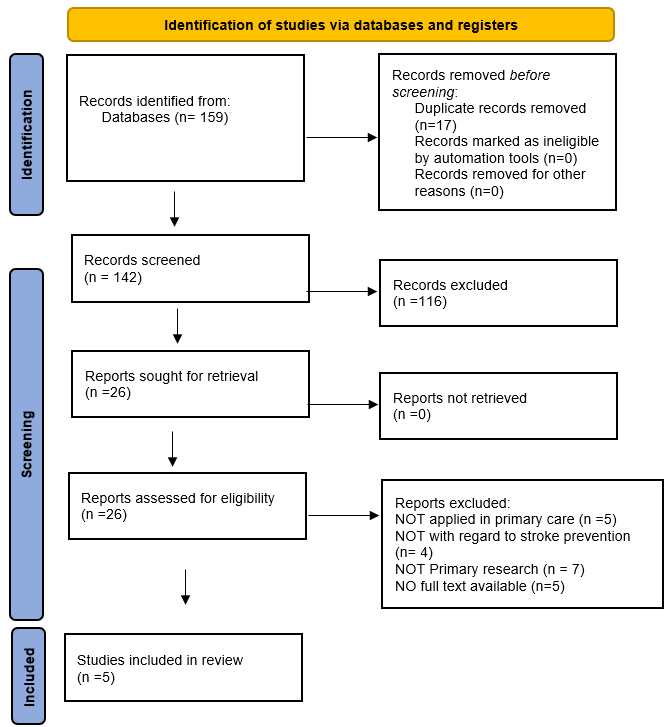
Quality Assessment QA (Risk of bias)
The default QA template in Covidence is Cochrane’s Risk of Bias (RoB) and was used with the Critical Appraisal Skills Program (CASP) tool to assess the methodological quality of the RCT papers selected for retrieval by two independent reviewers before they were included in the review. The AXIAL tool was used to assess the quality of the cross-sectional studies.
From the qualitative data, a thematic synthesis was be used to identify significant categories and themes. The evidence from the SR of qualitative research methods was used to determine how much confidence can be placed in qualitative finding.
Tables 3, 4, and 5 represent the results of this assessment. Four out of the five studies considered as high level of quality, the remain considered average. The major methodological limitations were the lack of groups similarity and the risk-benefits analysis of the studies.
Results
Characteristics of the Studies Included
A sum of 470,392 patients at a high risk of stroke were assessed in the five studies13-17 included in this systematic review. These patients were assessed in primary care settings distributed in various parts of the world, including the Netherlands (Arts et al., 2017), Sweden (Karlson et al., 2018), Italy (Mazzaglia et al., 2016), Denmark (Bonnevie et al., 2004), and Australia (Wang & Bajorek, 2016). Three of the studies were cluster randomized trials (Mazzaglia et al., 2016; Arts et al., 2017; Karlson et al., 2018), two were a cross-sectional studies (Wand et al., 2016; Bonnevie et al., 2004). The five studies had research investigations conducted in the years ranging between 2004 and 2018. Bonnevie et al. (2004) was the earliest study, while Karlson et al. (2018) were the latest. For additional details, see Table 2.
Core Aspects of the CDSS Tools
A typical CDSS tool should have three core aspects: the user interface, the processing layer or inference engine, and the data management layer, which acts as the system’s base18. The three aspects work collaboratively to complete the architecture of a CDSS tool. Clinical data, patient data, and the system’s knowledge algorithms are executed at the base. The inference engine then processes this information and relays it to the interface layer in the form of alerts, recommendations, and reminders 19The user interface can be accessed on any device; mobile messages, mobile applications, web, or on the dashboard of an EHR system. In the five studies, the recurrent aspect of the CDSS tools used was the integration capability of the CDSS and the EHR system of the institution where the study was set.
CDSS Tools Developed
Knowledge-based CDSSs
Knowledge-based and non-knowledge-based are common classifications for CDSS in healthcare. The CDSS in the reviewed studies are all knowledge-based, where in these systems, rules are developed (IF-THEN statements), with the system obtaining data to assess the rule and providing an action or result 20.
Karlsson et al. (2018) looked to establish whether the CDSS tool increases adherence to guidelines regarding the prevention of stroke in atrial fibrillation (AF) patients17. In this research, Cambio Healthcare Systems built a computerised tool integrated into EHR with primary care providers and cardiologists. This CDSS predicts AF, a key stroke risk factor, using EHR data. The CDSS alerts the doctor of a risk factor and suggests a treatment.
For Wang & Bajorek (2016), the CDSS model was meant to facilitate the prescription of two antithrombotics, warfarin or NOACs. Wang & Bajorek (2016) did use an improved Computerised Antithrombotic Risk Assessment Instrument 2.0 instead of an interactive tool (CARATV2.0)21. Wang & Bajorek (2017) discovered promising results in optimising antithrombotic treatment, they found that CARATV2.0 increases antithrombotic usage and lowers stroke risk. A significant increase in antithrombotic was experienced in the first application of the NAOCs CARATV2.0 22
Bonnevie et al. (2004) used the PRECARD® program. The model was designed to test the preventive abilities of the tool against cardiovascular risks using the Copenhagen Risk Score. The recommendations are based on sex, age, previous heart disease, a familial predisposition to heart disease, systolic blood pressure, diabetes, lifestyles like smoking, body mass index (BMI), and high-density lipoprotein (HDL) cholesterol14. Previously, Thomsen et al. (2001) had published the development research of the PRECARD® program and approved its probability of improving the clinical prevention of stroke23. Despite its ability to improve the quality of cardiological risk preventiveness in primary care, the tool has been the response for a 14 percent increase in waiting time 14
Similarly, Arts et al. (2017) developed a CDSS tool that drew patient data and based recommendations on the Dutch general practitioners’ guidelines for atrial fibrillation. This CDSS tool was implemented to be non-obtrusive to the general practitioner’s flow of activities to improve guidelines16. The increased effectiveness, in this case, is a result of non-interruptive recommendations and responses.
In the Italy-based study by Mazzaglia et al. (2016), the investigators used the Health Search Cegedim Strategic Data Longitudinal Patient Database (HS-CSD-LPD)15. It uses Health Search Network data to make recommendations to the medical practitioners involved in the study. The usage of HS-CSD-LPD has not been very widespread compared to other tools like the PRECARD® program and CARATV2.0.
The design of Mazzaglia et al. (2016) sought to ascertain the hypotheses that CDSS will increase the use of preventive therapies to cardiovascular therapies and, by so doing, reduce the dependency on drugs15.
Effectiveness of CDSS to Prevent Stroke
The general usefulness of CDSS is to ease the work of the physicians as well as lowering the risk of various health conditions. The risk of stroke has been assessed on the fronts of diagnosis, treatment, and prevention and can be monitored and greatly lowered through timely and computerized decision prompts14,21.
CDSS and Stroke Screening
CDSS tools have demonstrated abilities to lower the risk of stroke by monitoring most risk factors. However, Mazzaglia et al. (2016) found that the system's number of alerts sent is so large that it had to be discontinued from screening. Despite such decisions poking holes in the logic of useability, the larger health informatics literature can agree that such alerts cannot be ignored unless the functionality is not in use ()24. Karlsson et al. (2018) standardized the CHA2DS2-VASc tool to activate a patient with AF diagnosis17. The tool was, however, not very useful in diagnostics, despite relaying the risk traits of stroke, a CDSS cannot provide a certain diagnostic.
CDSS and Clinical Guidelines Adherence
Clinical guidance adherence was assessed by two of the included studies (Arts et al., 2017; Karlsson et al., 2018)16,17. Notably, the use of CHA2DS2-VASc by Karlsson et al. (2018) produced significant results indicating adherence differences after 12 months of follow-up between groups using the tool and the control. The difference in findings between the CDSS group and the control was (73.0%, 95% CI 64.6%–81.4%) versus (71.2%, 95% CI 60.8%–81.6%) p = 0.013. Echoing these results, Arts et al. 2017 reported a 10 to 20 percent absolute effect size and adherence rate of the randomized groups in the study whose prompts are ignored with a reason; and whose prompts are ignored without reason). In a subsequent study, Arts et al. (2017) could not establish a significant difference between the interventional (55 percent) and control (50 percent) groups in terms of sticking to the therapeutic prompts16,17.
Discussion
The use of Clinical Decision Support Systems (CDSS) in stroke prevention has the potential to bring about significant benefits to patient outcomes. These benefits include enhanced accuracy and efficiency in identifying high-risk patients, improved adherence to evidence-based guidelines for stroke prevention, and earlier diagnosis and intervention. However, the implementation of CDSS in clinical practice is not without potential risks. This paper explores the possible downsides of CDSS in stroke prevention, including the increased workload for healthcare providers, potential loss of personal interaction between providers and patients, and the risk of overreliance on the CDSS, leading to overdiagnosis or overtreatment.
In an effort to enhance the pharmacological management of high-risk cardiovascular patients in primary care, Mazzaglia (2016) created CDSS. The findings of their study were encouraging, demonstrating that the implementation of CDSS led to a positive impact on the quality of care for diabetic patients15.
Wang (2016) developed a decision support tool called the Computerized Antithrombotic Risk Assessment Tool version 2.0 (CARATV2.0) to help doctors decide whether to use novel oral anticoagulants (NOACs) or warfarin to prevent strokes in older patients with AF21. While the tool improved the use of antithrombotic treatment, it also led to more frequent prescription of blood thinners than what general practitioners typically do. This suggests the potential for overtreatment, where patients receive unnecessary or excessive treatment, and highlights the importance of optimizing treatment utilization to prevent potential harm to patients.
In terms of adherence to clinical guidelines, Karlsson et al. (2018) discovered that integrating a clinical decision support (CDS) tool for stroke prevention into the EHR has the potential to enhance adherence to stroke prevention guidelines for patients with AF. AF is associated with significant morbidity, especially in stroke patients. Karlsson et al. (2018) demonstrated that the use of a CDS could improve adherence to guidelines for anticoagulant therapy in patients with AF. The automated CDS tool integrated into EHRs functioned by producing an alert for AF patients at risk of stroke if they did not receive appropriate treatment. This intervention is straightforward and ultimately increased the utilization of anticoagulants in patients with atrial fibrillation. Nevertheless, Karlsson et al. (2018) noted that the difference is small in comparison to cases where the CDS tool was not used, the capability of CDS cannot be underestimated17. It actually indicates the potential for further enhancements that might lead to compounding beneficial effects on patient care.
Bonnevie et al. (2005) conduct a study on the implementation of a program (PRECARD®) used for electronic cardiovascular disease risk assessment and management by general practitioners in Denmark14. They not only access its usage but also attitudes towards the program. It was reported that by using the program, there was a favourable effect on the interaction between general practitioners and patients. Even so, there were some general practitioners that were reported to have stopped using the program citing reasons such as technical problems and incompatibility of routines with the program.
Despite the potential benefits of CDSS in stroke prevention, studies such as that conducted by Arts et al. (2017) have shown that CDSS are not always effective in increasing adherence to clinical guidelines. Specifically, the study found no significant difference in guideline adherence between patients where a CDSS was used and control groups. Interestingly, both the intervention and control groups showed an increase in guideline adherence during the trial period. In light of these findings, Arts et al. (2017) recommend that future efforts to implement CDSS in clinical practice should focus on improving multi-domain CDSS to address challenges in implementation and facilitate their effectiveness in real-world settings16.
Implications
The findings from various studies on the effectiveness of CDSS in preventing first stroke in primary care are inconclusive, highlighting the need for further research in this area. While some studies have shown promising benefits, the variability in CDSS characteristics and the heterogeneity of results indicate that more investigations are necessary to draw stronger conclusions.
Therefore, future studies should focus on identifying ways to improve CDSS effectiveness in preventing stroke, particularly by exploring the pre-implementation and implementation processes and the human factor, which can play crucial roles in determining the effectiveness of CDSS in real-world settings. Given the potential for CDSS to enhance stroke prevention in primary care, it is important to continue studying and improving the effectiveness of these systems.
This systematic review carries significant implications as it underscores the potential benefits of CDSS in preventing stroke in primary care, emphasizing the possibility of substantial advantages that such systems may offer and their ability to enhance the communication and collaboration between patients and general practitioners.
Limitations
The scarcity of data stemming from the analyzed studies impedes the formation of definitive conclusions regarding the efficacy of CDSS in preventing stroke in primary care. Furthermore, the presence of heterogeneous results, characterized by varying degrees of beneficial outcomes, underscores the urgency of additional investigations aimed at attaining more consistent and robust results. Additionally, the absence of pre- and post-intervention measurements to evaluate the role of CDSS in stroke prevention poses another obstacle that necessitates further exploration in subsequent studies.
Conclusion
CDSS can improve primary care outcomes and prevent disease occurrence. While most interventions achieve only small to moderate improvements in patient outcomes, some studies demonstrate the potential benefits of CDSS in preventing strokes and enhancing primary care. However, designing reliable CDSS remains a challenge. Future research should explore new ways of designing such systems to ensure reliability.
Acknowledgments
We thank Walsh Mary, senior library assistant at Kings College London for her assistance and support in refining the search strategy. Salha Alasiri is supported by King Faisal University through the Saudi Cultural Bureau in London.
Supplementary Tables
Table 1: Search Strategy Table
Database
|
Search Strategy
|
Ovid (Medline and Embase)
|
Clinical? Decision? Support? Systems
Or
Computerized Decision? Support? Tools
Or
CDSS
AND
prevent
AND
Stroke
AND
Primary care
|
Science Direct
|
TS=(“Clinical? decision? support? systems” OR
“Algorithm” OR decision OR tool)” OR
AND
TS= “Prevention”
TS= Stroke
TS= primary care
|
Cinahl
|
Clinical decision support systems
Decision Support Systems, Clinical
CDSS
TX prevent
TX Stroke
TX Primary care
|
Table 2: Studies characteristic
Lead Author, Year: Yishen Wang MBBS, 2016
Study Title: Clinical pre-test of a computerised antithrombotic risk assessment tool for stroke prevention in atrial fibrillation patients: giving consideration to NOACs
Country: Australia
Aim of Study: The study wanted to pre-test a CDSS tool that would help clinicians in selecting antithrombotics.
Study Design:A cross-sectional study
Setting: 369 general practice patients with atrial fibrillation (AF) participating in the previous study (2012) were involved here. Their age was ≥65 years with a confirmed diagnosis of AF New South Wales. Their information was available in the former study database.
Participants Characteristics: 393 patients with AF [mean age 78.0 (±7.0) years], 54.5% were male and 45.8% (n = 180) were aged ≥80 years.
Type of CDSS Tools Utilized: An updated version of CARATV2.0 based on latest clinical evidence.
Outcomes: CARATV2.0 recommended warfarin for 360 (91.6%) patients, NOAC for 5 (1.3%) patients, either rivaroxaban or apixaban for 6 (1.5%) patients, andapixaban for 9 (2.2%). This was in the case where warfarin was recommended as first-line therapy.
Lead Author, Year: Lise Bonnevie, 2004
Study Title: The use of computerized decision support systems in preventive cardiology-principal results from the national PRECARD@ survey in Denmark
Country: Denmark
Aim of Study: Conduct a cardiovascular disease risk assessment and management in Danish patients since 1999 using the PRECARD® program.
Study Design: A cross-sectional study
Setting: 3568 general practitioners registered in Denmark were contacted. An online survey conducted on 592 general practitioners in Denmark.
Participants Characteristics: 400/3568 took the postal survey and 291/400(73%) responded. The participants were subdivided into users 60(22%) [males 45(75%)] and mean age 49.7 years, ex-user 28(10%) [males 18(64%)] and mean age 51 years, and never user 191(68%) [males 126(66%)] and mean age 51.7 years.
Type of CDSS Tools Utilized: The PRECARD® program for CVD risk assessment and management.
Outcomes: 21.5% GPs use the program, 10% have used it before, and the program is utilized at a rate of 64% weekly. The usage of the program affects the patients favourably by enhancing the dialogue between them and the practitioner. However, it also prolongs consultation time.
Lead Author, Year: Lars O. Karlsson, 2018
Study Title: A clinical decision support tool for improving adherence to guidelines on anticoagulant therapy in patients with atrial fibrillation at risk of stroke: A cluster-randomized trial in a Swedish primary care setting (the CDS-AF study)
Country: Sweden
Aim of Study: To assess whether adherence to guidelines regarding the prevention of stroke can be increased by using a CDSS tool.
Study Design: A cluster randomized trial
Setting: 444,347 Swedish patients obtained from 43 primary care clinics county of Östergötland will be into CDSS and control groups for the randomized study.
Participants Characteristics: Patients with atrial fibrillation at risk of stroke, 43 primary care clinics in the county of Ö stergö tland, Sweden (population 444,347, patients with AF
Type of CDSS Tools Utilized: A CDSS embedded in a standard electronic health record (EHR) and uses medical record data to identify patients with AF and one or more risk factors who have not yet been prescribed anticoagulant medication, according to the CHA2DS2-VASc algorithm.
Outcomes: CARATV2.0 suggested any NOAC for 279 (70.9%) patients, rivaroxaban or apixaban for 80 (20.4%) patients, apixaban for 9 (2.3%) patients, and warfarin for 12 (3.1%) patients. This was in the case of where NAOCs were recommended as first-line therapy.
Lead Author, Year: Giampiero Mazzaglia, 2016
Study Title: Effects of a computerized decision support system in improving pharmacological management in high-risk cardiovascular patients: A cluster-randomized open-label controlled trial.
Country: Italy
Aim of Study: Testing if using CDSS can favourably affect the prevalence of preventive therapies according to the recommendation guidelines and check whether the number of days of drug interactions will reduce among patients with a high risk of cardiovascular diseases.
Study Design: A cluster randomized controlled trial
Setting: 197 general practitioners were randomly assigned to groups that will either receive alerting computerized decision support system integrated into standard software (intervention arm) or the standard software alone (control arm)
Participants Characteristics: Diabetic patients, 21230 patients with diabetes, 3956 with acute myocardial infarction, and 2158 with stroke were analysed, 197 Italian general practitioners, high-risk cardiovascular patients.
Type of CDSS Tools Utilized: A CDSS embedded in a standard EHR and uses medical record data to identify patients with AF and one or more risk factors who have not yet been prescribed anticoagulant medication, according to the CHA2DS2-VASc algorithm.
Outcomes: For 279 (70.9%) patients, CARATV2.0 recommended any NOAC, rivaroxaban or apixaban for 80 (20.4%) patients, apixaban for 9 (2.3%) patients, and warfarin for 12 (3.1%) patients. When NAOCs were suggested as first-line therapy, this was the situation.
Lead Author, Year: Derk L. Arts, 2017
Study Title: Effectiveness and usage of a decision support system to improve stroke prevention in general practice: A cluster randomized controlled trial
Country: The Netherlands
Aim of Study: Using a non-obtrusive CDSS integrated into the workflow to increase guideline adherence. Also, to figure out why people don't follow guidelines.
Study Design: A cluster randomized controlled trial
Setting: A randomized control experiment on the Dutch general practices. To prevent contamination bias, randomization was done at the GP practice level. The allocation ratios were 2:1:1 and 1:1:1. The 'sample' function was used to generate a random sequence from the list of GP practices provided by DA in the statistical environment R. GPs were aware that they were assigned to a system variant, but they were unaware of their assignment and how the variants differed.
Participants Characteristics: 781 patients were included randomized into post-study control (259 patients) [mean age(SD): 73.73 (14.7)] and post-study intervention (522 patients) [mean age(SD): 72.79 (12.61).
Type of CDSS Tools Utilized: Real-time, non-interruptive, and based on data from electronic health records were all attributes positively associated with effectiveness when a decision support system was established. The recommendations were based on the CHA2DS2-VAsc for stroke risk stratification, which is used in the Dutch general practitioners guideline for atrial fibrillation.
Outcomes: There was a decreased utilization of the system (5%) which dropped over time. There was a 58% rate of dismissal and 42% rate of acceptance to notifications (76 were responded to). Acceptance had improved in both groups by a factor of 8% and 5% but the difference was not significant between the analysed groups.
Table 4: The CASP assessment table below represents the appraisal of three RCTs included
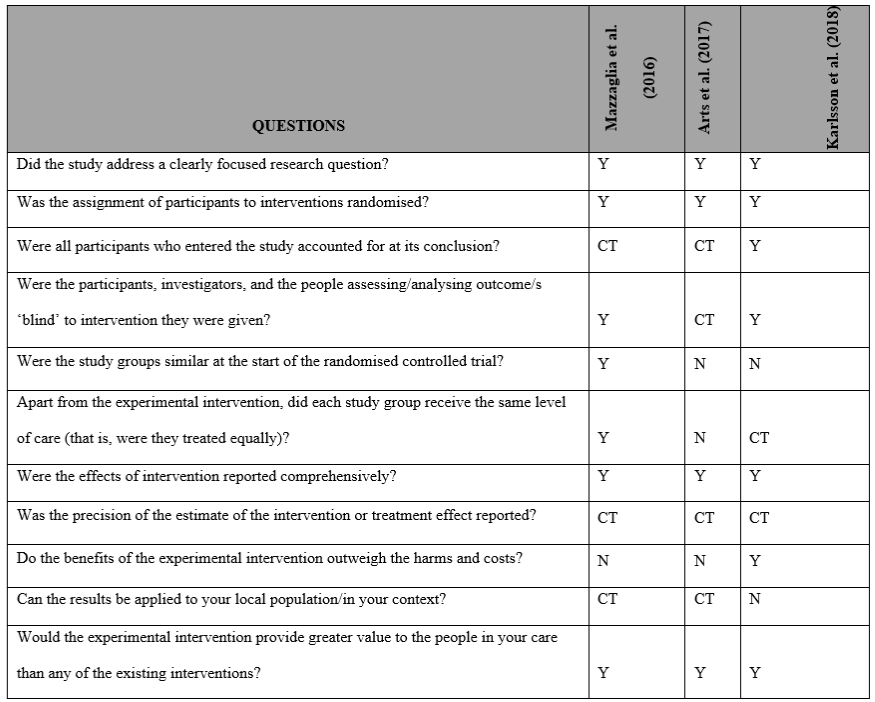
Table 5: The AXIAL assessment table below represents the appraisal of all the two cross-sectional studies included
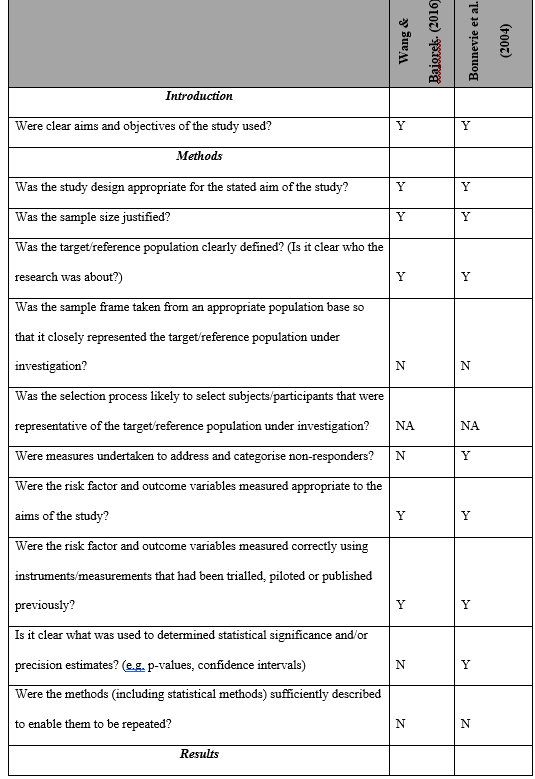
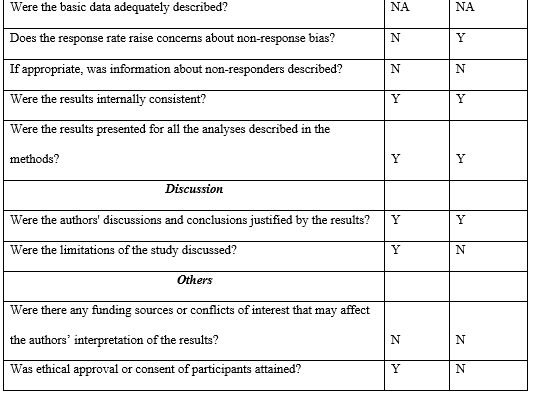
References
1. Pusic M, Ansermino JM. Clinical decision support systems. BCMJ. 2004;46(5):236–239.
2. Anooj, P. K. (2012). Clinical decision support system: Risk level prediction of heart disease using weighted fuzzy rules. Journal of King Saud University-Computer and Information Sciences, 24(1), 27-40.
3. Zikos D, DeLellis N. CDSS-RM: a clinical decision support system reference model. BMC Med Res Methodol. 2018 Nov 16;18(1):137. doi: 10.1186/s12874-018-0587-6.
4. Shemeikka T, Bastholm-Rahmner P, Elinder CG, Vég A, Törnqvist E, Cornelius B et al. A health record integrated clinical decision support system to support prescriptions of pharmaceutical drugs in patients with reduced renal function: design, development and proof of concept. Int J Med Inform. 2015;84:387-95.
5. Matricardi S, Farello G, Operto FF, Coppola G, Verrotti A. What are the challenges with the pharmacological management of epilepsy in patients with Attention Deficit Hyperactivity Disorder (ADHD)? Exp Opin Pharmacother. (2020) 21:737–9. 10.1080/14656566.2020.1732351
6. Saini, V., Guada, L., & Yavagal, D. R. (2021). Global epidemiology of stroke and access to acute ischemic stroke interventions. Neurology, 97(20 Supplement 2), S6-S16.
7. Feigin VL, Norrving B, Mensah GA. Global Burden of Stroke. Circ Res. 2017 Feb 3;120(3):439-448. doi: 10.1161/CIRCRESAHA.116.308413.
8. Thapa, L., Shrestha, S., Kandu, R., Ghimire, M. R., Ghimire, S., Chaudhary, N. K., Pahari, B., Bhattarai, S., Kharel, G., Paudel, R., Jalan, P., Chandra, A., Phuyal, S., Adhikari, B., Aryal, N., & Kurmi, O. P. (2021a). Prevalence of stroke and stroke risk factors in a south-western community of Nepal. Journal of Stroke and Cerebrovascular Diseases, 30(5), 105716. https://doi.org/10.1016/j.jstrokecerebrovasdis.2021.105716
9. Feigin VL, Brainin M, Norrving B, et al. What is the best mix of population-wide and high-risk targeted strategies of primary stroke and cardiovascular disease prevention? J Am Heart Assoc. 2020;9: e014494
10. Feigin VL, Brainin M, Norrving B, et al. World Stroke Organization (WSO): global stroke fact sheet 2022. Int J Stroke 2022; 17: 18–29.
11. Bright, T. J., Wong, A., Dhurjati, R., Bristow, E., Bastian, L., Coeytaux, R. R., ... & Lobach, D. (2012). Effect of clinical decision-support systems: a systematic review. Annals of internal medicine, 157(1), 29-43.
12. Njie, G. J., Proia, K. K., Thota, A. B., Finnie, R. K., Hopkins, D. P., Banks, S. M., ... & Community Preventive Services Task Force. (2015). Clinical decision support systems and prevention: a community guide cardiovascular disease systematic review. American journal of preventive medicine, 49(5), 784-795.
13. Wang, Y., & Bajorek, B. (2016). Clinical pre‐test of a computerised antithrombotic risk assessment tool for stroke prevention in atrial fibrillation patients: giving consideration to NOACs. Journal of Evaluation in Clinical Practice, 22(6), 896-902.
14. Bonnevie, L., Thomsen, T., & Jørgensen, T. (2005). The use of computerized decision support systems in preventive cardiology—principal results from the national PRECARD® survey in Denmark. European Journal of Cardiovascular Prevention & Rehabilitation, 12(1), 52-55.
15. Mazzaglia, G., Piccinni, C., Filippi, A., Sini, G., Lapi, F., Sessa, E., ... & Caputi, A. P. (2016). Effects of a computerized decision support system in improving pharmacological management in high-risk cardiovascular patients: A cluster-randomized open-label controlled trial. Health informatics journal, 22(2), 232-247.
16. Arts, D. L., Abu-Hanna, A., Medlock, S. K., & van Weert, H. C. (2017). Effectiveness and usage of a decision support system to improve stroke prevention in general practice: a cluster randomized controlled trial. PLoS One, 12(2), e0170974.
17. Karlsson, L. O., Nilsson, S., Bång, M., Nilsson, L., Charitakis, E., & Janzon, M. (2018). A clinical decision support tool for improving adherence to guidelines on anticoagulant therapy in patients with atrial fibrillation at risk of stroke: a cluster-randomized trial in a Swedish primary care setting (the CDS-AF study). PLoS medicine, 15(3), e1002528.
18. Sutton, R. T., Pincock, D., Baumgart, D. C., Sadowski, D. C., Fedorak, R. N., & Kroeker, K. I. (2020). An overview of clinical decision support systems: benefits, risks, and strategies for success. NPJ digital medicine, 3(1), 1-10.
19. Wasylewicz, A. T. M., & Scheepers-Hoeks, A. M. J. W. (2019). Clinical decision support systems. Fundamentals of clinical data science, 153-169.
20. Berner, E.S. and La Lande, T.J. (2007) “Overview of clinical decision support systems,” Health Informatics, pp. 3–22. Available at: https://doi.org/10.1007/978-0-387-38319-4_1.
21. Wang V, Hsieh CC, Huang YL, Chen CP, Hsieh YT, Chao TH. Different utilization of intensive care services (ICSs) for patients dying of hemorrhagic and ischemic stroke, a hospital-based survey. Medicine (Baltimore). (2018) 97:e0017. 10.1097/MD.0000000000010017 بدل 21 لو ما
22. Wang Y, Bajorek B. Selecting antithrombotic therapy for stroke prevention in atrial fibrillation: Health professionals' feedback on a decision support tool. Health Informatics J. 2018 Sep;24(3):309-322. doi: 10.1177/1460458216675498.
23. Thomsen, T. F., Davidsen, M., Ibsen, H., Jørgensen, T., Jensen, G., & Borch-Johnsen, K. (2001). A new method for CHD prediction and prevention based on regional risk scores and randomized clinical trials; PRECARD® and the Copenhagen Risk Score. European Journal of Cardiovascular Prevention & Rehabilitation, 8(5), 291-297.
24. Van Der Sijs H, Mulder A, Van Gelder T, et al. Drug safety alert generation and overriding in a large Dutch university medical centre. Pharmacoepidemiol Drug Saf 2009; 18: 941–947.
Author Biographies
Salha Fayea Alasiri (salha.alasiri@kcl.ac.uk) is a PhD Candidate at School of Population Health And Environmental Sciences, Kings College London, and a Lecturer at College of Applied Medical Sciences, King Faisal University
Abdel Douiri (abdel.douiri@kcl.ac.uk) is a Reader in Medical Statistics, at School of Population Health And Environmental Sciences, Kings College London.
Saffanah Altukistani (Saffanah.alturkistani.16@ucl.ac.uk) is a PhD Candidate at Institute of Epidemiology & Health, University College London, as well as a teaching assistant at Umm Al-Qura University.
Talay Porat (t.porat@imperial.ac.uk) is a Senior Lecturer at Faculty of Engineering, Dyson School of Design Engineering, Imperial College London.
Ola Mousa, PhD (olaessam1977@yahoo.com) is currently an associate professor at King Faisal University, College of Applied Medical Sciences- Nursing Department, Saudi Arabia. Her area of interest in research is Maternity Nursing, Women and Adolescent Health, and mothers of children with special needs. Presently, she is teaching at King Faisal University, Kingdom of Saudi Arabia, the chairperson of the Community Engagement Committee, and deputy of the students' activity committee. She is a supporter of students and cares for their needs as a chairperson of the Guidance and Skills Development Unit.