Introduction
Nationwide initiatives designed to improve the efficiency, safety, and quality of the delivery of healthcare are driving the adoption of interoperable health information exchange (HIE). In June 2014, the Office of the National Coordinator for Health Information Technology (ONC) released its 10-year vision to achieve an interoperable health information technology (IT) infrastructure,1 which identifies patient matching as part of its three-year agenda. In the vision statement, the ONC reported that it “will also address critical issues such as data provenance, data quality and reliability, and patient matching to improve the quality of interoperability, and therefore facilitate an increased quantity of information movement.”2
This growing demand for HIE brings the challenges of accurate patient identification to the forefront. A nationwide patient data matching strategy will facilitate patient matching and provide the foundation for interoperable HIE. This goal can be accomplished with the standardization of primary and secondary data elements, and adoption of a uniform data capture methodology.
Lack of a standard data set can lead to patient records not being linked to one another in the HIE, resulting in an incomplete health record being available to the provider for the patient being treated, thereby defeating the purpose of the HIE. Even more concerning is the potential for different patients being identified as the same, resulting in the possibility of improper care rendered on the basis of inaccurate patient information. In addition to patient care concerns, sharing inaccurate information also poses the risk of privacy breaches and erodes consumer confidence in the benefits of HIE. Errors in patient matching will only be compounded as healthcare organizations contend with advances in technology and the development and expansion of the eHealth Exchange (formerly known as the Nationwide Health Information Network).
Background
Historically, patient matching has been important within organizations to help identify duplicate medical records. When master patient indexes moved from paper to electronic, organizations gave little thought to data exchange, data formatting, or how data is entered into a person management system. In the past, data elements collected within a person management system were primarily used for billing purposes. Another challenge in the US healthcare system is that names are not unique and often change during a person’s lifetime or are presented differently. For example, Rob or Robert can never be used to identify a patient except in conjunction with more reliable information. One of the largest unresolved issues in the safe and secure electronic exchange of health information is a nationwide patient data matching strategy that would ensure the accurate, timely, and efficient matching of patients with their healthcare data across different systems and settings of care.3
Traditionally, patient matching has been done by health information management (HIM) professionals who manually review possible duplicate patients and manually update paper and electronic systems as needed. Manual review will not be sustainable in the future because electronic health records (EHRs) have created a vast amount of data that puts an undue budgetary burden on the HIE to employ additional staff responsible for ensuring data integrity. Currently, organizations are matching patient records within their own system but face challenges in incorporating patient matching techniques across care settings and different EHR systems.
As health IT innovation and system interoperability needs continue to grow, ensuring that patient data are accurate will be a key concern of many healthcare providers. Patients are taking charge of their healthcare and choosing to see different healthcare providers to address their conditions. This trend results in an increased need for organizations to share data, but the lack of a patient matching standard has prevented successful exchange. A patient match error could result in significant patient safety events, corrupt an organization’s medical records, and put lives at risk. It is paramount that organizations seek to establish a real-time automated patient matching process. For HIE to be successful, standards for data capture, definitions, and formatting must be developed to allow an electronic system to accurately identify patients across disparate EHR systems.
A nationwide patient data matching strategy will assist in matching patient records in the HIE, as well as improve clinical care delivery, decrease the cost of duplicative diagnostic tests, link clinical results, provide accurate data for analytics, underpin research efforts, and establish a foundation for patient-centric care delivery. Standardization is also needed at the source of the data because individual healthcare organizations have different patient naming conventions, use different methods for identifying duplicate patient records in their own systems, and may have multiple records for a patient within their own EHR systems. When all EHR systems capture and store patient demographic elements in the same format, algorithms will be able to match patient records consistently within and across healthcare organizations. Regardless of which algorithm is used, healthcare organizations’ use of consistent standards for patient identification will facilitate accurate patient matching.4
The adoption of a nationwide patient matching strategy that standardizes a set of patient demographic elements stored in a standard format would support existing models of patient matching such as the federated identity knowledge discovery model5 and the centralized identity knowledge approach.6
Standardization of Primary and Secondary Data Elements
One of the most common solutions for patient matching has been to create a unique patient identifier. This identifier could consist of a single identifying element or use multiple standardized elements that would take a single form for all patients. A single patient’s health information may be stored and identified through the use of multiple identifiers within a healthcare organization or across multiple organizations. Healthcare organizations and HIEs rely on the use of key primary and secondary demographic data elements available within unique systems to successfully link patient records.
Many HIEs have adopted patient identification approaches that use a unique identifier data element to establish identification within the boundaries of the HIE itself. In this approach, the HIE assigns a unique patient identifier (UPI) within the HIE and uses that identifier for patient matching purposes. A UPI can be provided to a patient by a regulatory body or authority. This approach has long been one of the most contentious issues in healthcare privacy because of uncertainty as to who provides and maintains control of the patient identifier. Many of the current HIE architecture designs revolve around control being placed into the hands of the HIE. This approach, although effective at the local level, creates a process that is out of alignment with national interoperability initiatives. The creation of local HIE patient matching architectures has generally not been successful in the United States because of the contention over the use of a universal patient identifier.
Existing standards that are widely accepted in the marketplace, such as the United States Postal Service (USPS) address definitions and the Council for Affordable Quality Healthcare (CAQH) and Uniform Hospital Discharge Data Set definitions, provide a means to normalize data across disparate systems. Increasing the data elements utilized and incorporating standard data definitions into technical requirements for person capture provides a solid foundation regardless of the algorithm. Instituting a standard format and accepted definitions for data element capture minimizes the burden on staffing in routine business operations, providing long term financial relief. Standardizing data element capture across the market will affect vendors financially and result in some time constraints in EHR architecture building. However, the positive results in accurate patient matching and successful interoperable HIE are of greater consideration.
Embracing standardized data attributes, requiring minimal primary data capture, and increasing the use of secondary data elements will provide a solid foundation for interoperability with patient linking. Figure 1 highlights recommended primary and secondary data attributes that will facilitate accurate patient matching. Appendix A outlines these primary and secondary data attributes in further detail with references to Health Level 7 (HL-7), Accredited Standards Committee X12 (ASC X12), and CAQH standards and recommendations from organizations including the National Committee on Vital and Health Statistics, the Healthcare Information and Management Systems Society (HIMSS), the ONC, the Office of Management and Budget, and the USPS.
Adoption of Sophisticated Patient Matching Algorithms and Integration Profiles
A fundamental and critical success factor for HIE is the ability to accurately link multiple records for the same patient across the disparate systems of the participating organizations. Algorithms can support many of the patient matching functions envisioned in HIE. In this approach, mathematical calculations and predefined rules are applied to pairs of patient records to facilitate matching of patient identifiers. Basic algorithms that compare selected data elements, such as name, date of birth, and gender, are the simplest technique for matching records. Intermediate algorithms use more advanced techniques to compare and match records by assigning subjective weights to demographic elements for use in a scoring system to determine the probability of matching patient records. Advanced algorithms contain the most sophisticated set of tools for matching records and rely on mathematical theory and statistical models to determine the likelihood of a match.
Two primary types of algorithms can be used to determine matching patient records: deterministic and statistical/mathematical algorithms. Deterministic record matching programs compare values in various fields to determine whether the values are an exact match or a partial match to the value of that field in another record. The primary challenge with this type of algorithm is that data elements must be exact for a match to be recognized, and any variation in elements is considered nonmatching, resulting in many false negatives and duplicate patient records. Structured values (such as gender, race, or marital status) can help facilitate patient matching with a deterministic algorithm, but the process becomes more challenging when dealing with variations in free-text elements, such as a person’s name, or when demographics may have been captured incorrectly, such as an incorrect number in a patient’s date of birth or Social Security number. Statistical/mathematical algorithms assign weights to near matches of data elements and then determine the probability of a match between the patient records. Thousands of different algorithms use statistical and mathematical constructs for patient record matching, and advanced algorithms often utilize a combination of many different algorithms.
Policies that are designed to support capturing demographics in a standardized format can also facilitate patient matching. The process of capturing data is an operational consideration that cannot be taken lightly. In this case, standards such as those developed by CAQH can assist because they provide rules that define how a patient’s name is captured and exchanged. See Appendix B for a sample naming convention policy that provides structure for data entry where free text is required.
Although patient matching algorithms have been widely adopted, methods of matching patient records within and across organizations have not been adopted uniformly across the industry. No consensus exists regarding patient matching accuracy thresholds, and each organization employs its own matching algorithm and patient matching methods, resulting in inconsistent results across the industry. Standards development organizations have developed integration profiles to resolve several algorithm issues related to patient matching. One of the most well-known of these profiles is the Integrating the Health Enterprise (IHE) Cross-Community Patient Discovery (XCPD) profile, which allows for Patient Identification (PIX) and Patient Demographic Query (PDQ) transactions to be conducted to facilitate patient matching across multiple organizations within a single HIE. The XCPD profile has seen widespread adoption as a standard for query-based exchange of patient records, and, in addition to a patient matching algorithm, XCPD and/or PIX and PDQ transactions can be used to help link multiple patient identities within or across healthcare communities.
Conclusion
Without accurate patient matching, providers may have incomplete information on their patients or may be presented with inaccurate information. A nationwide patient identification standard will facilitate patient matching and provide the foundation for interoperable HIE. This goal can be accomplished with the standardization of the following:
- Primary and secondary data elements;
- The use of industry-recognized data definitions;
- Elimination of free text, except for the name; and
- Separate data entry for data elements.
A common set of standardized data elements to be used across multiple interoperability standards is ideal to support accurate patient matching. While the organizational impact of increased data entry is a consideration, the capture of additional data elements enables significant improvement of patient linking accuracy until a unique patient identifier becomes available or biometric technology improves, providing a more cost-effective matching method. Secondary data recommendations increase matching probability in the pediatric population and also serve as an additional level for data triangulation in the adult population. Data integrity improves with the elimination of free text and the utilization of national data standards. Free-text entry is necessary for patient names, but capture of the complete legal name in discrete fields minimizes data entry errors.
Common data capture of demographic elements through uniform policies that are widely shared will help to overcome the policy variations across organizations and appropriately manage the free-text component of data entry for names. Continued use and adoption of existing technical profiles supports varying query and retrieval approaches for patient demographic data by providing flexibility to allow the use of various combinations where they are most feasible and applicable.
Standardizing data capture through the use of existing national standards, increasing the number of primary data elements, and incorporating secondary data elements will provide a means to accurately identify participants in HIE. The glossary of recommended primary and secondary data elements in Appendix A and the sample patient naming policy in Appendix B can be used to ensure consistency of data elements and provide structure for data entry where free text is required.
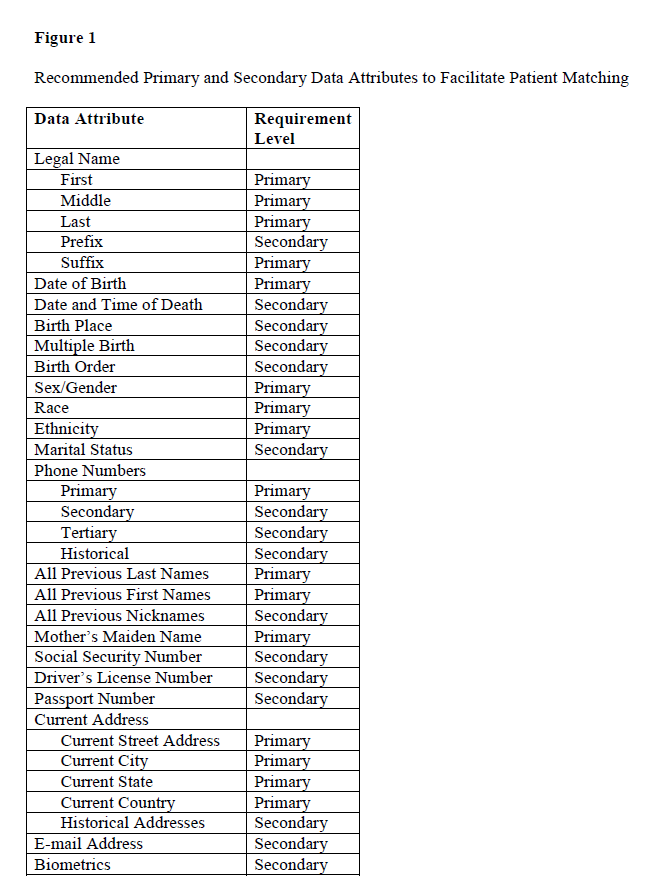
Acknowledgments
The authors thank Patricia Buttner, RHIA, CDIP, CCS; Melanie Endicott, MBA/HCM, RHIA, CDIP, CCS, CCS-P, FAHIMA; Beth Just, MBA, RHIA, FAHIMA; Annessa Kirby; Harry B. Rhodes, MBA, RHIA, CHPS, CDIP, CPHIMS, FAHIMA; and Sheldon H. Wolf.
Notes
- Office of the National Coordinator for Health Information Technology. Connecting Health and Care for the Nation: A 10-Year Vision to Achieve an Interoperable Health IT Infrastructure. Available at http://healthit.gov/sites/default/files/ONC10yearInteroperabilityConceptPaper.pdf.
- Ibid., p. 6.
- Stevens, Lee, and Kate Black. “Patient Matching Findings Released.” Health IT Buzz. February 21, 2014. Available at http://www.healthit.gov/buzz-blog/electronic-health-and-medical-records/patient-matching-findings-released/.
- Office of the National Coordinator for Health Information Technology. Connecting Health and Care for the Nation: A 10-Year Vision to Achieve an Interoperable Health IT Infrastructure.
- Integrating the Healthcare Enterprise (IHE). IHE IT Infrastructure (ITI) Technical Framework Supplement 2009-2010: Cross-Community Access (XCA). August 10, 2009. Available at http://www.ihe.net/Technical_Framework/upload/IHE_ITI_TF_Supplement_Cross_Community_Access_XCA_TI_2009-08-10.pdf.
- Mussi, José, Nathan Domeij, Karen Wiiting, and Charles Parisot. IHE IT Infrastructure XDS Patient Identity Management White Paper. Integrating the Healthcare Enterprise (IHE). March 4, 2011. Available at http://www.ihe.net/Technical_Framework/upload/IHE_ITI_WhitePaper_Patient_ID_Management_Rev2-0_2011-03-04.pdf.
References
American Health Information Management Association (AHIMA). “Managing the Integrity of Patient Identity in Health Information Exchange (Updated).” Journal of AHIMA 85, no. 5 (2014): 60–65. Available at http://library.ahima.org/xpedio/groups/public/documents/ahima/bok1_050658.hcsp?dDocName=bok1_050658.
Bipartisan Policy Center. Challenges and Strategies for Accurately Matching Patients to Their Health Data. June 2012. Available at http://bipartisanpolicy.org/library/challenges-and-strategies-accurately-matching-patients-their-health-data/.
Dimitropoulos, Linda. Privacy and Security Solutions for Interoperable Health Information Exchange: Perspectives on Patient Matching: Approaches, Findings, and Challenges. Chicago, IL: RTI International, June 30, 2009. Available at http://www.healthit.gov/sites/default/files/patient-matching-white-paper-final-2.pdf.
Healthcare Information and Management Systems Society (HIMSS). Patient Identity Integrity Toolkit: Model Interface Protocols. January 2012. Available at https://www.himss.org/files/HIMSSorg/content/files/PrivacySecurity/PII02_Interface_Protocols.pdf.
HIMSS. Patient Identity Integrity Toolkit: Model Data Practices. September 2011. Available at http://www.himss.org/files/HIMSSorg/content/files/piitoolkit/PIIModelDataPractices.pdf.
Moehrke, John. “Healthcare Security/Privacy: Patient Identity Matching.” Healthcare Security/Privacy. December 7, 2011. Available at http://healthcaresecprivacy.blogspot.com/2011/12/patient-identity-matching.html.
Office of the National Coordinator for Health Information Technology (ONC). Patient Identification and Matching Final Report. By Genevieve Morris, Greg Farnum, Scott Afzal, Carol Robinson, Jan Greene, and Chris Coughlin. Baltimore, MD: Audacious Inquiry, LLC, February 7, 2014. Available at http://www.healthit.gov/sites/default/files/patient_identification_matching_final_report.pdf.
Purkis, Ben, Genevieve Morris, Scott Afzal, Mrinal Bhasker, and David Finney. Master Data Management within HIE Infrastructures: A Focus on Master Patient Indexing Approaches. Audacious Inquiry, LLC, prepared for the Office of the National Coordinator for Health Information Technology, September 30, 2012. Available at http://www.healthit.gov/sites/default/files/master_data_management_final.pdf.
Author Biographies
Katherine G. Lusk, MHSM, RHIA, is the chief health information management and exchange officer at Children’s Health System of Texas in Dallas, TX.
Neysa Noreen, RHIA, is a data integrity and applications manager at Children’s Hospitals and Clinics of Minnesota in Minneapolis, MN.
Godwin Okafor, RHIA, FAC-P/PM, FAC-COR, is a Program Analyst at the Department of Veterans Affairs Central Office in Washington, DC.
Kimberly Peterson, MHIM, RHIA, CHTS-TS, is a clinical application analyst at Children’s Hospital Colorado in Aurora, CO.
Erik Pupo, MBA, CPHIMS, FHIMSS, is a specialist leader of federal health at Deloitte Consulting LLP in Arlington, VA.